Get started¶
This notebook contains the simple examples of time series forecasting pipeline using ETNA library.
Table of Contents
1. Creating TSDataset¶
Let’s load and look at the dataset
[1]:
import pandas as pd
[2]:
original_df = pd.read_csv("data/monthly-australian-wine-sales.csv")
original_df.head()
[2]:
month | sales | |
---|---|---|
0 | 1980-01-01 | 15136 |
1 | 1980-02-01 | 16733 |
2 | 1980-03-01 | 20016 |
3 | 1980-04-01 | 17708 |
4 | 1980-05-01 | 18019 |
etna_ts is strict about data format: * column we want to predict should be called target
* column with datatime data should be called timestamp
* because etna is always ready to work with multiple time series, column segment
is also compulsory
Our library works with the special data structure TSDataset. So, before starting anything, we need to convert the classical DataFrame to TSDataset.
Let’s rename first
[3]:
original_df["timestamp"] = pd.to_datetime(original_df["month"])
original_df["target"] = original_df["sales"]
original_df.drop(columns=["month", "sales"], inplace=True)
original_df["segment"] = "main"
original_df.head()
[3]:
timestamp | target | segment | |
---|---|---|---|
0 | 1980-01-01 | 15136 | main |
1 | 1980-02-01 | 16733 | main |
2 | 1980-03-01 | 20016 | main |
3 | 1980-04-01 | 17708 | main |
4 | 1980-05-01 | 18019 | main |
Time to convert to TSDataset!
To do this, we initially need to convert the classical DataFrame to the special format.
[4]:
from etna.datasets.tsdataset import TSDataset
[5]:
df = TSDataset.to_dataset(original_df)
df.head()
[5]:
segment | main |
---|---|
feature | target |
timestamp | |
1980-01-01 | 15136 |
1980-02-01 | 16733 |
1980-03-01 | 20016 |
1980-04-01 | 17708 |
1980-05-01 | 18019 |
Now we can construct the TSDataset.
Additionally to passing dataframe we should specify frequency of our data. In this case it is monthly data.
[6]:
ts = TSDataset(df, freq="1M")
/Users/an.alekseev/PycharmProjects/etna/etna/datasets/tsdataset.py:114: UserWarning: You probably set wrong freq. Discovered freq in you data is MS, you set 1M
warnings.warn(
Oups. Let’s fix that
[7]:
ts = TSDataset(df, freq="MS")
We can look at the basic information about the dataset
[8]:
ts.info()
<class 'etna.datasets.TSDataset'>
num_segments: 1
num_exogs: 0
num_regressors: 0
num_known_future: 0
freq: MS
start_timestamp end_timestamp length num_missing
segments
main 1980-01-01 1994-08-01 176 0
Or in DataFrame format
[9]:
ts.describe()
[9]:
start_timestamp | end_timestamp | length | num_missing | num_segments | num_exogs | num_regressors | num_known_future | freq | |
---|---|---|---|---|---|---|---|---|---|
segments | |||||||||
main | 1980-01-01 | 1994-08-01 | 176 | 0 | 1 | 0 | 0 | 0 | MS |
3. Forecasting single time series¶
Our library contains a wide range of different models for time series forecasting. Let’s look at some of them.
3.1 Simple forecast¶
Let’s predict the monthly values in 1994 in our dataset using the NaiveModel
[11]:
train_ts, test_ts = ts.train_test_split(train_start="1980-01-01",
train_end="1993-12-01",
test_start="1994-01-01",
test_end="1994-08-01")
[12]:
HORIZON = 8
from etna.models import NaiveModel
#Fit the model
model = NaiveModel(lag=12)
model.fit(train_ts)
#Make the forecast
future_ts = train_ts.make_future(HORIZON)
forecast_ts = model.forecast(future_ts)
Now let’s look at a metric and plot the prediction. All the methods already built-in in etna.
[13]:
from etna.metrics import SMAPE
[14]:
smape = SMAPE()
smape(y_true=test_ts, y_pred=forecast_ts)
[14]:
{'main': 11.492045838249387}
[15]:
from etna.analysis import plot_forecast
[16]:
plot_forecast(forecast_ts, test_ts, train_ts, n_train_samples=10)
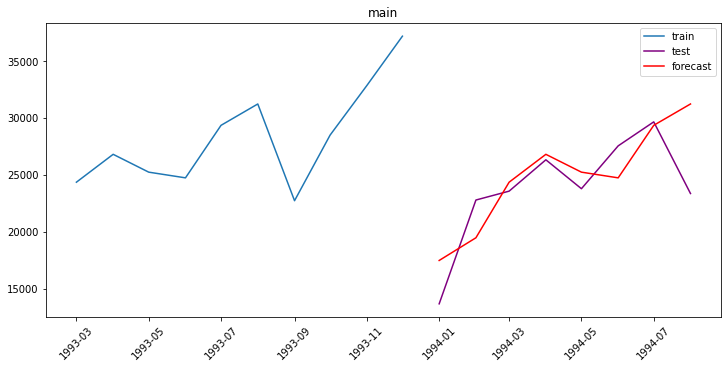
3.2 Prophet¶
Now try to improve the forecast and predict the values with Prophet.
[17]:
from etna.models import ProphetModel
model = ProphetModel()
model.fit(train_ts)
#Make the forecast
future_ts = train_ts.make_future(HORIZON)
forecast_ts = model.forecast(future_ts)
INFO:prophet:Disabling weekly seasonality. Run prophet with weekly_seasonality=True to override this.
INFO:prophet:Disabling daily seasonality. Run prophet with daily_seasonality=True to override this.
Initial log joint probability = -4.75778
Iter log prob ||dx|| ||grad|| alpha alpha0 # evals Notes
85 409.431 0.000868182 75.9007 1.007e-05 0.001 143 LS failed, Hessian reset
99 409.49 0.000113932 67.8321 1 1 160
Iter log prob ||dx|| ||grad|| alpha alpha0 # evals Notes
143 409.516 5.45099e-05 61.0448 7.513e-07 0.001 261 LS failed, Hessian reset
199 409.632 0.00045371 68.4983 0.3105 1 335
Iter log prob ||dx|| ||grad|| alpha alpha0 # evals Notes
222 409.725 0.000155328 49.7537 3.299e-06 0.001 403 LS failed, Hessian reset
290 409.763 5.21333e-06 74.9937 6.81e-08 0.001 543 LS failed, Hessian reset
299 409.763 1.85382e-07 50.4613 0.6115 0.6115 556
Iter log prob ||dx|| ||grad|| alpha alpha0 # evals Notes
349 409.766 3.2649e-05 65.1573 4.184e-07 0.001 674 LS failed, Hessian reset
385 409.767 3.20082e-07 70.9723 5.71e-09 0.001 772 LS failed, Hessian reset
398 409.767 1.71798e-08 62.1219 0.3754 1 790
Optimization terminated normally:
Convergence detected: relative gradient magnitude is below tolerance
[18]:
smape(y_true=test_ts, y_pred=forecast_ts)
[18]:
{'main': 10.626418322451338}
[19]:
plot_forecast(forecast_ts, test_ts, train_ts, n_train_samples=10)
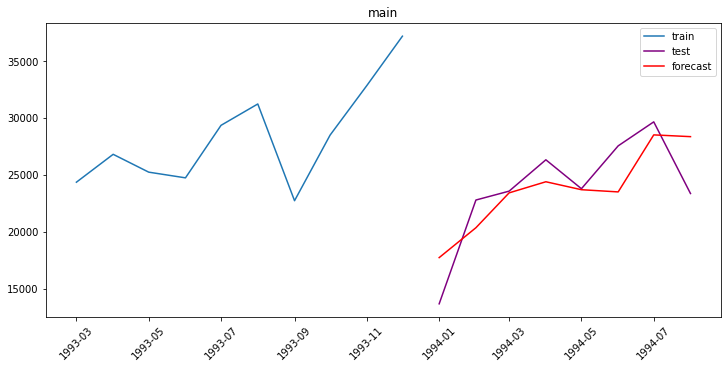
3.2 Catboost¶
And finally let’s try the Catboost model.
Also etna has wide range of transforms you may apply to your data.
Here how it is done:
[20]:
from etna.transforms import LagTransform
lags = LagTransform(in_column="target", lags=list(range(8, 24, 1)))
train_ts.fit_transform([lags])
[21]:
from etna.models import CatBoostModelMultiSegment
model = CatBoostModelMultiSegment()
model.fit(train_ts)
future_ts = train_ts.make_future(HORIZON)
forecast_ts = model.forecast(future_ts)
[22]:
from etna.metrics import SMAPE
smape = SMAPE()
smape(y_true=test_ts, y_pred=forecast_ts)
[22]:
{'main': 10.715432057450386}
[23]:
from etna.analysis import plot_forecast
train_ts.inverse_transform()
plot_forecast(forecast_ts, test_ts, train_ts, n_train_samples=10)
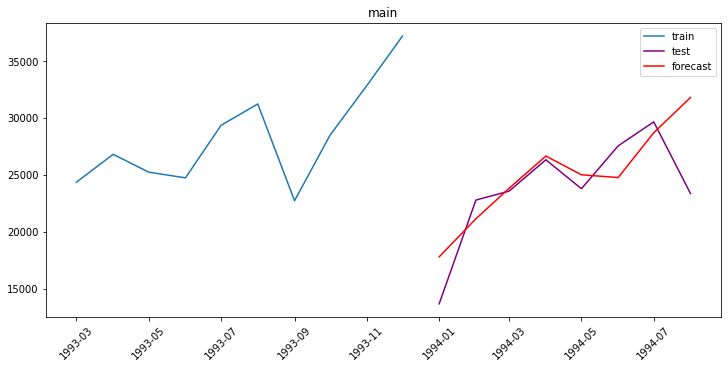
4. Forecasting multiple time series¶
In this section you may see example of how easily etna works with multiple time series and get acquainted with other transforms etna contains.
[24]:
original_df = pd.read_csv("data/example_dataset.csv")
original_df.head()
[24]:
timestamp | segment | target | |
---|---|---|---|
0 | 2019-01-01 | segment_a | 170 |
1 | 2019-01-02 | segment_a | 243 |
2 | 2019-01-03 | segment_a | 267 |
3 | 2019-01-04 | segment_a | 287 |
4 | 2019-01-05 | segment_a | 279 |
[25]:
df = TSDataset.to_dataset(original_df)
ts = TSDataset(df, freq="D")
ts.plot()
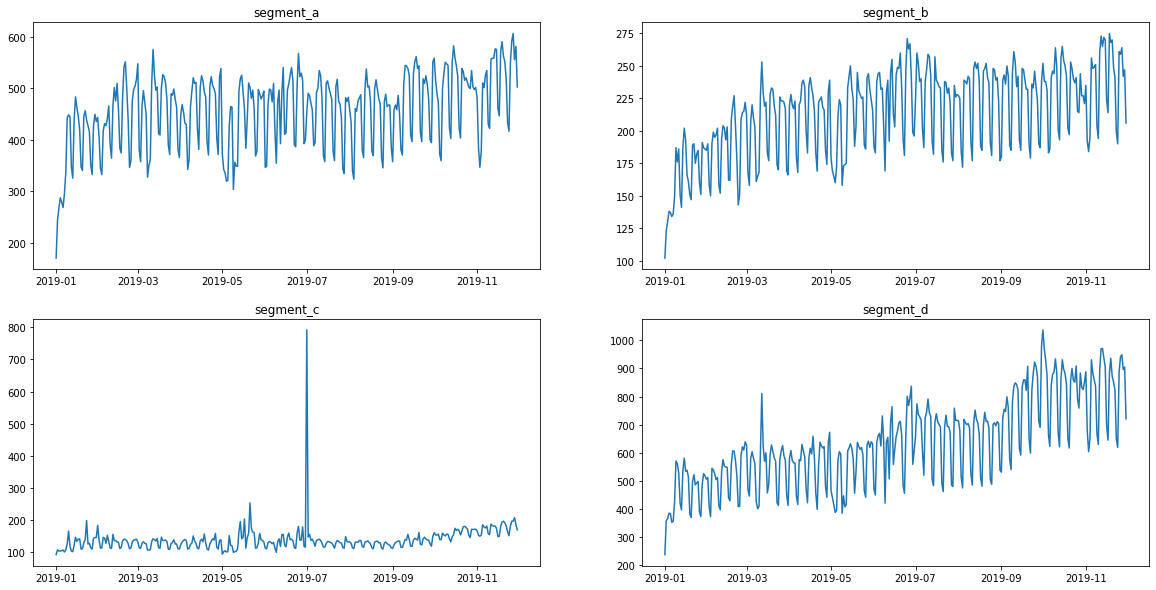
[26]:
ts.info()
<class 'etna.datasets.TSDataset'>
num_segments: 4
num_exogs: 0
num_regressors: 0
num_known_future: 0
freq: D
start_timestamp end_timestamp length num_missing
segments
segment_a 2019-01-01 2019-11-30 334 0
segment_b 2019-01-01 2019-11-30 334 0
segment_c 2019-01-01 2019-11-30 334 0
segment_d 2019-01-01 2019-11-30 334 0
[27]:
import warnings
from etna.transforms import MeanTransform, LagTransform, LogTransform, \
SegmentEncoderTransform, DateFlagsTransform, LinearTrendTransform
warnings.filterwarnings("ignore")
log = LogTransform(in_column="target")
trend = LinearTrendTransform(in_column="target")
seg = SegmentEncoderTransform()
lags = LagTransform(in_column="target", lags=list(range(30, 96, 1)))
d_flags = DateFlagsTransform(day_number_in_week=True,
day_number_in_month=True,
week_number_in_month=True,
week_number_in_year=True,
month_number_in_year=True,
year_number=True,
special_days_in_week=[5, 6])
mean30 = MeanTransform(in_column="target", window=30)
[28]:
HORIZON = 30
train_ts, test_ts = ts.train_test_split(train_start="2019-01-01",
train_end="2019-10-31",
test_start="2019-11-01",
test_end="2019-11-30")
train_ts.fit_transform([log, trend, lags, d_flags, seg, mean30])
[29]:
test_ts.info()
<class 'etna.datasets.TSDataset'>
num_segments: 4
num_exogs: 0
num_regressors: 0
num_known_future: 0
freq: D
start_timestamp end_timestamp length num_missing
segments
segment_a 2019-11-01 2019-11-30 30 0
segment_b 2019-11-01 2019-11-30 30 0
segment_c 2019-11-01 2019-11-30 30 0
segment_d 2019-11-01 2019-11-30 30 0
[30]:
from etna.models import CatBoostModelMultiSegment
model = CatBoostModelMultiSegment()
model.fit(train_ts)
future_ts = train_ts.make_future(HORIZON)
forecast_ts = model.forecast(future_ts)
[31]:
smape = SMAPE()
smape(y_true=test_ts, y_pred=forecast_ts)
[31]:
{'segment_a': 6.059390208724589,
'segment_d': 4.987840592553301,
'segment_b': 4.210896545479207,
'segment_c': 11.729007773459358}
[32]:
train_ts.inverse_transform()
plot_forecast(forecast_ts, test_ts, train_ts, n_train_samples=20)
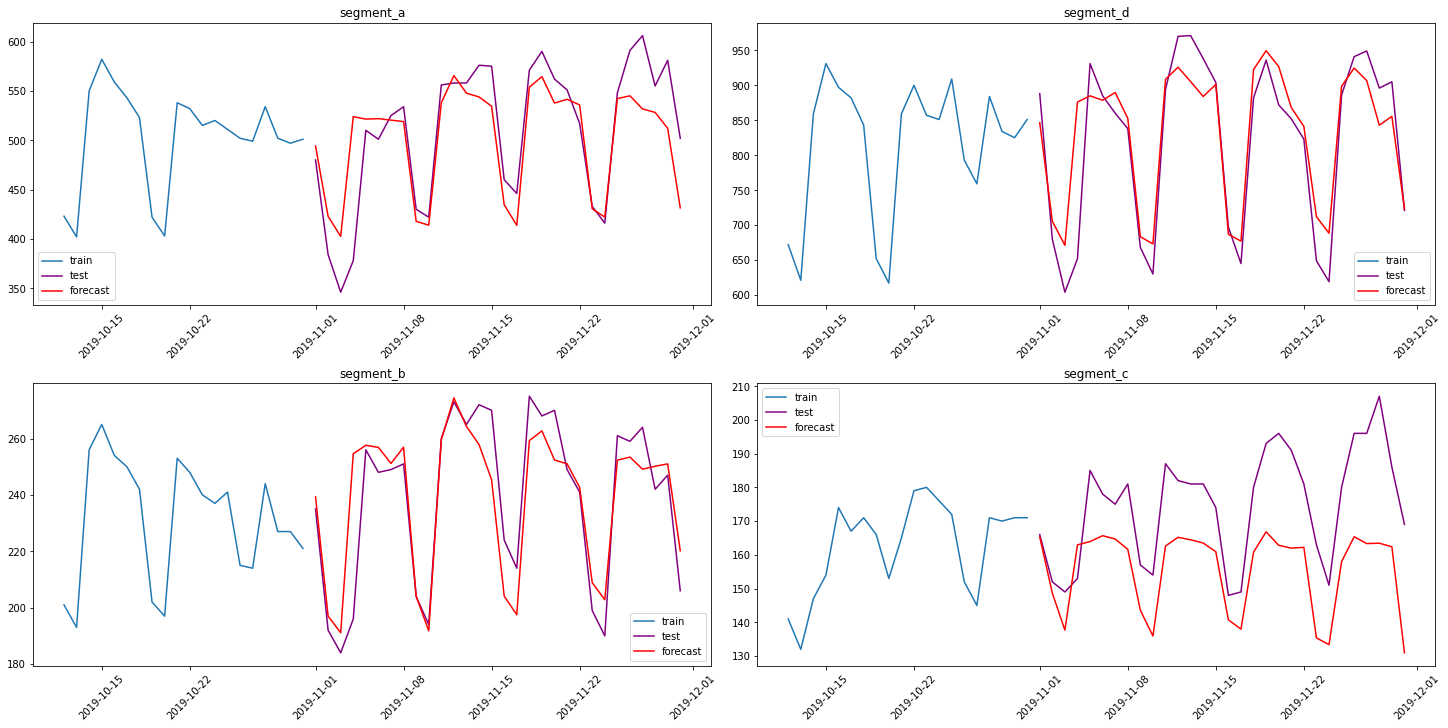
5. Pipeline¶
Let’s wrap everything into pipeline to create the end-to-end model from previous section.
[33]:
from etna.pipeline import Pipeline
[34]:
train_ts, test_ts = ts.train_test_split(train_start="2019-01-01",
train_end="2019-10-31",
test_start="2019-11-01",
test_end="2019-11-30")
We put: model, transforms and horizon in a single object, which has the similar interface with the model(fit/forecast)
[35]:
model = Pipeline(model=CatBoostModelMultiSegment(),
transforms=[log, trend, lags, d_flags, seg, mean30],
horizon=HORIZON)
model.fit(train_ts)
forecast_ts = model.forecast()
As in the previous section, let’s calculate the metrics and plot the forecast
[36]:
smape = SMAPE()
smape(y_true=test_ts, y_pred=forecast_ts)
[36]:
{'segment_a': 6.059390208724589,
'segment_d': 4.987840592553301,
'segment_b': 4.210896545479207,
'segment_c': 11.729007773459358}
[37]:
plot_forecast(forecast_ts, test_ts, train_ts, n_train_samples=20)
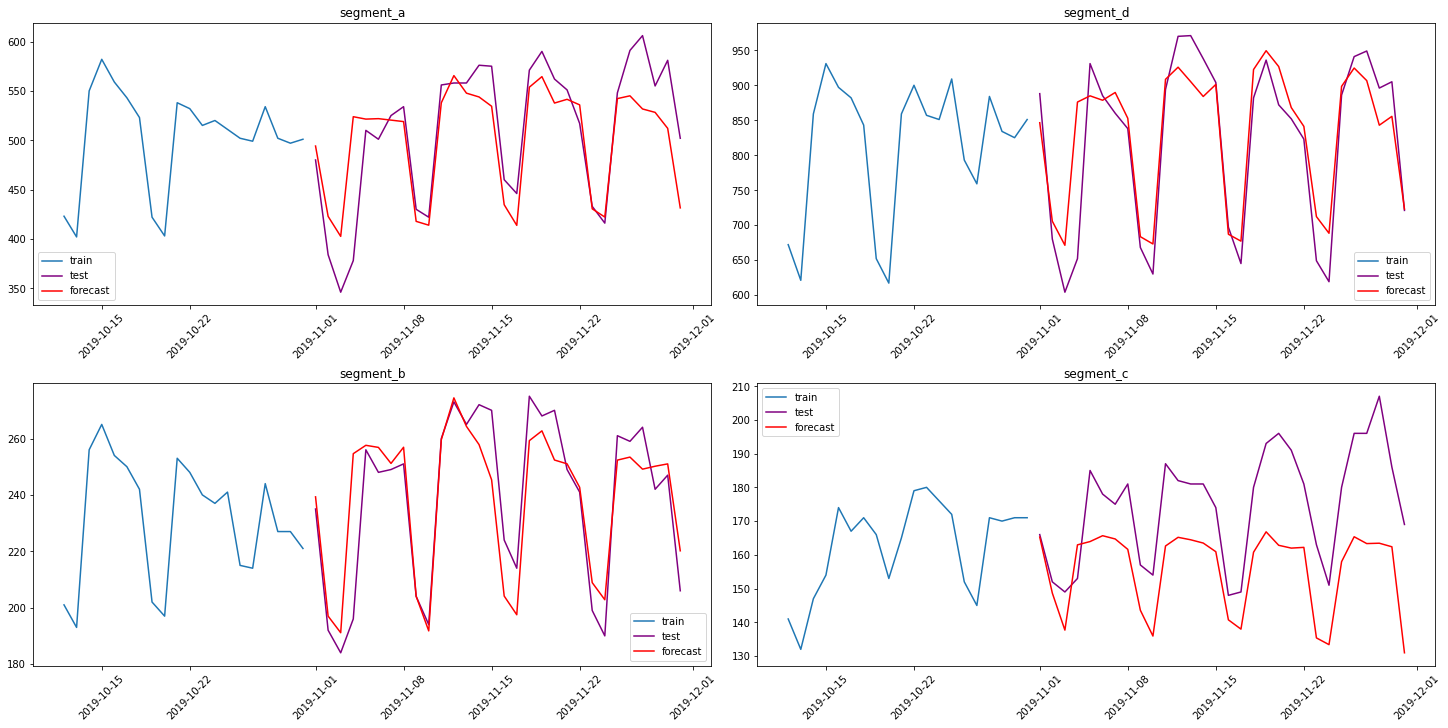